Building Blocks to Data and AI Literacy: A Step-by-Step Guide
In the ever-evolving landscape of modern business, data and AI literacy are becoming essential skills, a bit like mastering a global language. As we move towards more data-driven decision-making and AI integration, understanding how to effectively navigate this learning journey becomes crucial. Here’s how organizations can implement a structured, learning-by-doing approach to help employees become self-sufficient in utilizing data and AI. A good Data and AI Governance basis is also important and we wrote about that here.
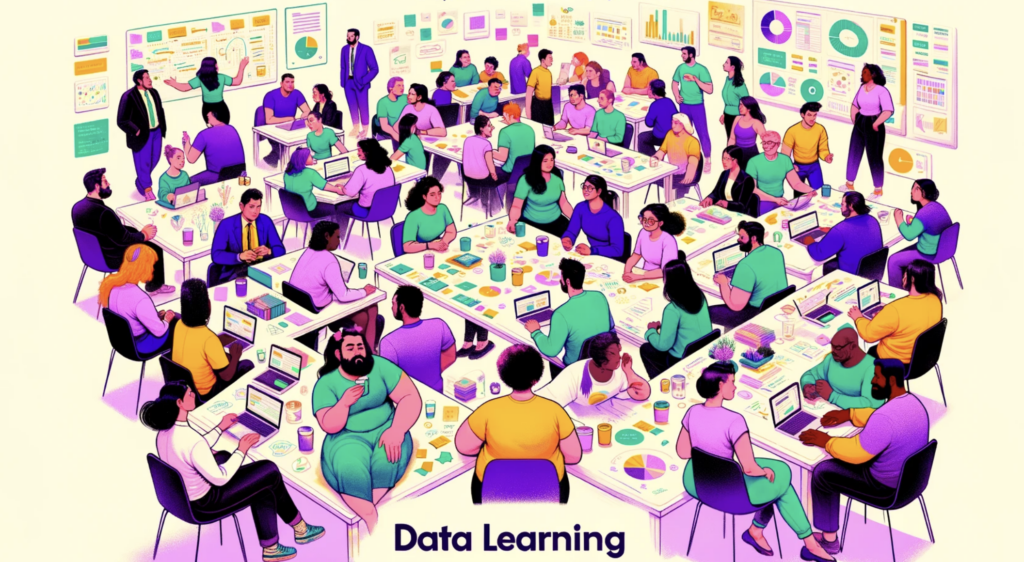
Understanding the Learning Stages People in Organizations go through
1. Beginner Level: Grasping the Basics
The journey begins with foundational knowledge. For data literacy, this includes understanding data types, basic data manipulation, and the significance of data in decision-making. For AI literacy, it involves an introduction to AI concepts, what AI can and cannot do, and real-world applications. At this stage, short, introductory sprints focused on key concepts help demystify complexities and lay the groundwork for more advanced learning.
2. Intermediate Level: Enhancing Skills through Application
Once the basics are understood, employees should start applying their knowledge to real-world scenarios. This could involve structured projects or challenges where learners manipulate datasets or build simple AI models relevant to their roles. This stage is crucial for reinforcing concepts and gaining confidence. Naturally understanding that not everyone will utilize data or AI on this level in their roles, but understanding the side of applying is important. Generative AI utilization is however another relevant application mode often in such cases. Organizations can support this through workshops, guided training sessions, and practical hands-on projects that encourage active learning. One example of this is the GOODIN and Splended Data Learning Sprint.
3. Advanced Level: Specializing and Innovating
As learners become more comfortable, they can move into specialized areas such as predictive analytics, machine learning, and advanced data visualization techniques for data literacy. For AI literacy, this might include deep learning, neural networks, or robotics. Advanced learners should engage in longer, more complex sprints that challenge their understanding and encourage innovation within their specific areas of interest. This demands a “fail fast” or “learning by doing” -type of learning culture in organizations and positive and encouraging management practices and commitment that enable failing for learning.
4. Expert Level: Leading and Mentoring
At the expert level, individuals are expected not only to be proficient but to lead initiatives and mentor others. They stay abreast of industry trends and continuously adapt to new technologies. Here, learning involves self-directed projects, leadership in sprints, and contributing to strategic decision-making with data-driven insights become real life value for business.
Implementing Learning by Doing: The Sprint Method
A learning sprint approach can be highly effective in progressing through these stages. Each sprint focuses on a specific skill or project, encouraging rapid learning and application. Here’s how it can work:
- Define Clear Objectives: Each sprint has specific, measurable goals to ensure focus and alignment with business objectives.
- Time-bound Challenges: Limit sprints to a few weeks to maintain urgency and engagement.
- Cross-functional Teams: Include employees from different departments to foster diverse perspectives and collaborative problem-solving.
- Reflect and Iterate: At the end of each sprint, gather feedback and reflect on lessons learned to improve the next cycle.
Supporting the Journey
Supporting employees through this journey requires more than just providing educational resources; it involves creating an ecosystem that promotes continuous learning and application. This includes access to the latest tools and technologies, opportunities for peer learning, and a culture that celebrates experimentation and learning from failure.
By implementing a structured, step-by-step approach to data and AI literacy, organizations can empower their teams to be not just participants but drivers of the data and AI revolution.
As they become more fluent, they will be able to leverage these skills to innovate and lead in their respective fields, ensuring the organization stays competitive in a data-driven future making sure the human drives the AI and not vice versa.